In a recent blog post, AI safety expert Ajeya Cotra argues that progress in artificial intelligence has been drive—and will continue to be driven—by both scale and schlep.
Scale (as you might infer from its name) can be thought of as what goes into an AI: the size and speed of the model, the quantity of training data used to build it, etc. It’s the machine’s raw computational power.
Schlep, by contrast, is how the model is used and packaged: the prompts we give it, the user interface, etc. It’s improving performance by working smarter, not harder.1
Small updates in schlep can vastly improve AI results. As Cotra notes, GPT-3.5’s rapid acquisition of 100 million users occurred only after it was integrated into a chat interface: “The difference between the two products was essentially presentation, user experience, and marketing.” Similarly, asking a Large Language Model to think step-by-step increases its accuracy in solving identical math problems:
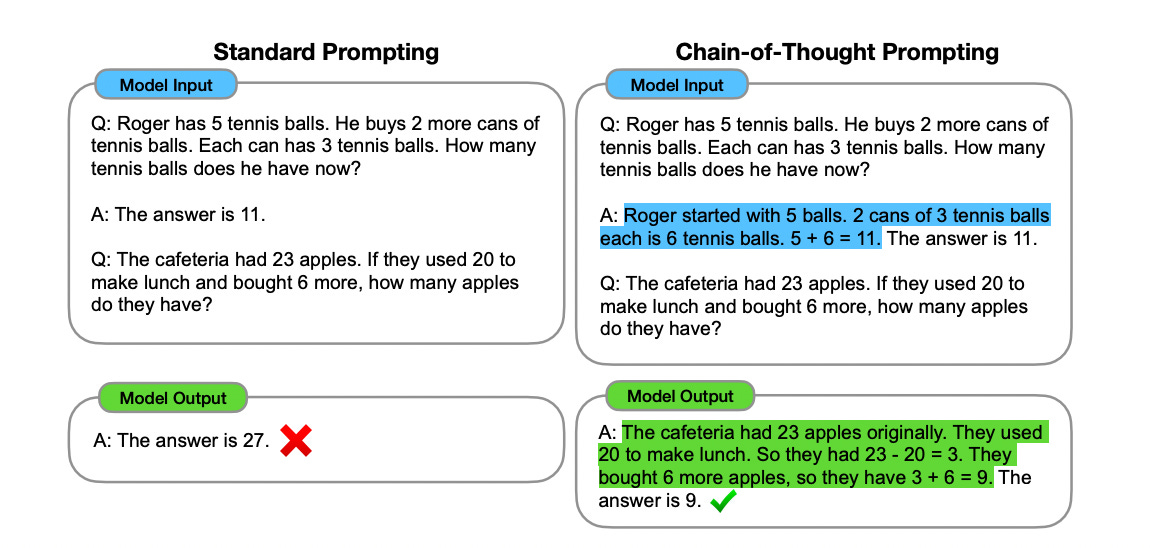
To further illustrate the distinction between scale and schlep, Cotra offers the analogy of engines vs. engine-powered cars:
If language models are like engines, then language model systems would be like cars and motorcycles and jet planes. Systems like Khan Academy’s one-on-one math tutor or Stripe’s interactive developer docs would not be possible to build without good language models, just as cars wouldn’t be possible without engines. But making these products a reality also involves doing a lot of schlep to pull together the “raw” language model with other key ingredients, getting them all to work well together, and putting them in a usable package.
Again, scale is the basic what that powers the technology; schlep is how it’s packaged.
I also find it illuminating to think about scale and schlep in the context of two other dominant metaphors for AI development: human learning, and natural selection.
In the case of human learning, scale can be thought of as raw ability/IQ and schlep can be thought of as “soft skills”/EQ.2 Most of us know people who are exceptionally smart or talented yet lack effectiveness because of other hard-to-see roadblocks. (Perhaps they struggle with mental health, analysis paralysis, communicating their ideas, or any number of psychosocial barriers.)
Similarly, it’s not hard to find examples of individuals who, despite having no measurable performance edge are wildly effective. Again the reasons for this are multiple and varied (charisma, work ethic, good instincts, etc.). I would even go so far as to say that above a certain threshold of ability, it’s more often schleppy factors that distinguish success than scale-y ones. Beyonce doesn’t have the most Grammys simply because she is the best vocalist; Tom Brady certainly isn’t the GOAT of quarterbacks simply because he is the most athletic.
In the natural selection metaphor, building and training Large Language Models is akin to choosing the “mutations” in the model that make it more “fit.” This creates an evolutionary pressure both for bigger AIs (i.e., scale) and better performance from AIs at any given size (i.e., schlep).
Again, with this analogy, we observe that schleppy factors are much more important than one might assume from the outset; brain size improves species’ fitness, yes—but there’s hardly a perfect correlation between number of neurons and “intelligent” behavior. Dogs have a comparable (or even smaller) brain size than wolves, yet are infinitely more trainable. Geese and monarch butterflies display similarly impressive navigational abilities in migration, yet birds presumably have far greater cognitive resources than insects. Brain size is not the end of the story.
Taken together, I don’t know if these metaphors necessarily means schlep is more important than scale—but they certainly suggest schlep is more neglected than scale.
More speculatively, I find it intuitive that improving AI schlep is more alignment-friendly than improving AI scale. To return to Cotra’s engine/car analogy: If you retrofitted your sedan with a jet engine, it seems more likely that something would go terribly wrong than if you simply designed a better car on top of existing engine technology—say, by adding a “crab mode”:
Is it possible that adding crab mode to a vehicle could cause unforeseen problems? Absolutely. I wouldn’t want to be in a car that accidentally crab-ified on the highway. But I think that on net, I’d feel much safer in a car equipped with untested new design features than one with an untested engine several orders of magnitude more powerful than any car before it.
So too with AI.
Of course the usual caveats are in order: It’s both/and, not either/or. No analogy is perfect—and all are subject to metaphor creep. I have no great technical ability in this domain.
But let me venture once again: Great technical ability will not carry the day in AI—certainly not on its own.
In this respect, I actually think the term schlep—which implies laborious effort—is a bit counterintuitive. Cotra’s examples are less suggestive of strain than of strategic improvement aimed at greater model efficacy or ease of use. (Of course, I’m all for Yiddish-based alliteration—perhaps “scale and shtick” or “scale and shpiel”?)
You might object that the line between these kinds of ability can be blurry, but I suspect the same is true of scale and schlep. (In the car analogy: If better engine design produces a more powerful engine, is that scale or schlep?)